This conversation with the composer Philip Glass and me discusses an exciting project in partnership with OpenAi, in which we trained a neural net on a corpus of Glass’ work. He offers commentary on the music created by “his AI”, as well as insights on composition and creating art. We then talk about the different limitations and capacities of humans and Artificial Intelligence–if and how neural nets can help us create art, appreciate art, and find the same things humans find meaningful. Due to the covid-19 pandemic, this call took place over video conference in December 2020.
Art and tech are both captivating to me because they frame the elevation and the limitations of being human. Art is also closely intertwined with technological advancements, as movement shifting art seems predicated on tech. For example, the photography of Martin Munkacsi from the 1920s and 1930s revolutionized the art, as he is often credited for being the first photographer to explore dynamic and candid styles. The emergence and ability of these new forms of creation coincided with the technological advancements at the time that enabled flash and faster shutters–candid and spontaneous movement shots wouldn’t have been technically possible to make with the cameras that existed before. The advancements in machine learning today, likewise, excite me for the possibilities and new forms in art and creation.
The goal of this project is to explore the capacities of artificial intelligence as a new medium (or instrument or tool?) for art, and to create a collaborative music composition with Philip Glass and “his AI.” More details about the project can be found below.
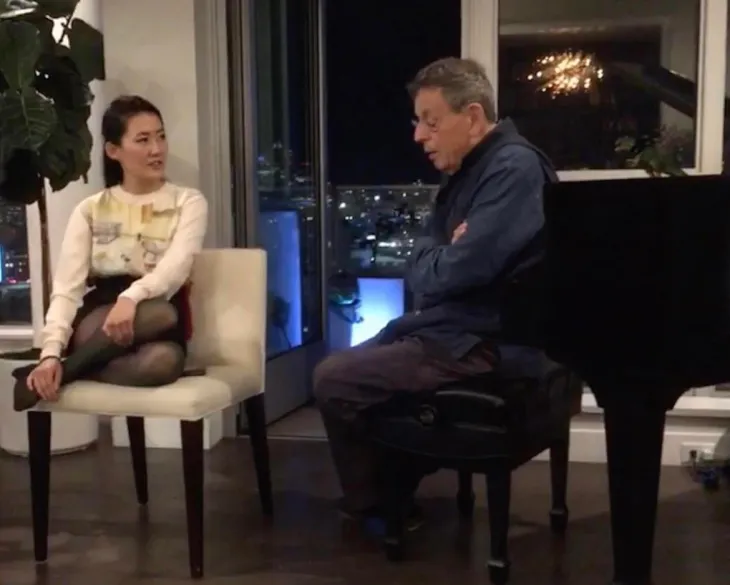
TRANSCRIPT
Philip: Nice to see you. I got the (music). Whose work is this?
Audrey: This is from the OpenAI Neural Net–
Philip: What was the music written for?
Audrey: It doesn’t have anything particular in mind. It’s not a movie score or–it didn’t have any intention like that since we weren’t able to set that…this wasn’t edited by any humans. It was entirely produced by the neural net.
Philip: Okay. My first…The music is okay. The structure of it is not well thought out. The first, the opening part, which is like the main theme–we never actually hear it again. That’s okay. You don’t have to do it that way, but that’s, maybe some of the best music is at the beginning. So why wouldn’t you want to hear it again? Some of the other parts, the more rhythmic parts, they’re okay, but that’s not where the core of the piece is. What I would suggest is go back to it and figure out is there an E theme, a B theme, a C theme, you know, what are the parts of it? The best, the most interesting part was at the beginning. After that, you kind of waited to hear if you could hear that again, and you never do.
Audrey: Which measure did you think–
Philip: Oh, I’m talking at the beginning…the first nine or ten measures. Well, I think the shape of it is–the kind of harmony and all that’s very familiar. That’s okay. That’s all fine. The shape of it is not well thought out, but that’s always the hardest thing.
Audrey: How do you mean shape?
Philip: Let’s say you had an A theme, right? And then you had a B theme. And if you analyze this, there are a lot of ideas here, but they’re not being presented in a way that are favorable to the eye to the piece. For example, that whole ending, the parts [measures] 21 through to the end. Nothing is coming in there. You know, the easiest thing to do , and would work–If when you got through to [measure] 51, if you went and did the beginning again. The machine doesn’t think structurally as well as the human brain does. I understand what you’re saying, “The machine did it,” but what does the machine know? You know, the machine doesn’t have memory–when we hear a piece of music, we hear parts of it and sometimes we want to hear that part again. And a lot of the structure — When we structure a piece we use sometimes like an A section; B section; C section, D section and we know what that is. The machine doesn’t know the difference between them.
What I am impressed with is the fact that the machine doesn’t make judgments that we make. We may say, “Oh, that’s a nice part. Can I hear that again?” The machine doesn’t do that, but as a composer, you do do that. The weakness of the piece is that no one is listening to it. But this is a very important part. This is the difference between machines and people. We have memory, we have preferences. We say, “I’d like to hear that again.” Machine doesn’t make judgments like that. All the machine is doing is putting out variations of what it did at the beginning–that in itself will not make a piece of music satisfying. What makes it satisfying is the way we hear it and the way we remember it and what we anticipate and when we hear the themes, the major themes, do we hear them again.
Look, it’s the same if we were looking at a dance piece, we would analyze it the same way, right? If you listen, or if you read a poem, you’ll analyze it in a similar way. The emotional content and the structure are connected.
Something is coming out of this that is important for you to know, which is that, the way we listen and the way we experience things is our ability to recall them and to understand them and to have preferences for them.
The thing about machines–it doesn’t do that. Now, you might be able to program that into a machine, but the question is whether the machine makes the same preferences that a human would and the answer is probably no. But this is a very interesting experience for me to hear this and say, “Oh, what’s wrong with it?” What’s wrong with it is no one’s listening to it. Now, there you have it. This is the difference between art and, let’s say, a bunch of ideas that don’t have an emotional direction to them.
Now what that tells us is that if, let’s say, that you take this and say, “Oh, well, there’s some stuff here. I can make a piece out of this.” Yes, that’s true. But that hasn’t happened yet. What this is is the first draft of a composer’s work, which is not a bad way of working. You’ll write down all the things you can think of, and then you’ve got material. It’s like the clothes in the closet. You say, “Oh, do I have any shirts? Do I have the right things? Or do I have the right colors?” Are they all there? They’re all there, yeah. But that doesn’t mean that when you put them on you’re wearing them in the right way. So there are two things: there’s content and then there’s the way we evaluate and experience the content.
Now, the machine can be very helpful, but what you’re showing me, it shows me the limits of the machine. I actually, to tell you the truth, I don’t really know–how do you tell a machine to listen the way a human does? I’m not sure. That’s the question, but what you can do, let’s say that you can say, “Oh, I like this part. I like this part. I like this part. I don’t need to hear those parts so many times. Okay. Let’s get rid of that.” You could take this piece, this music, you could turn it into an interesting piece. But that’s called composing. That’s where the composing comes in. This is an important thing to learn–that just putting together things that sound good is not going to work. It’s like going into the closet and putting on clothes without looking in the mirror and you go on and say, “That’s kind of interesting maybe, or maybe not.”
One of the reasons that we get our music training…we learn the Classics first. You learn the Bach, the Mozart and the Brahms and all that stuff. But then a little bit later, you’re doing some Stravinsky and then you say, “Oh, Stravinsky did that too. He must have studied that same music.” What Stravinsky was good at was taking abstract pieces and making sense of it. That’s what made him a good composer.
Writing these pieces, that’s okay, but it’s what you do with them. So I think this is on the way to becoming a piece of music, but you’re going to have to get involved with it a little bit more than that. The machine may not have that training, if I may put it that way. Now I don’t know enough about machine writing, but maybe there’s a way for a machine to be taught how to find something interesting. I don’t know. But you know, one of the problems we’ve always had with machine music is that it doesn’t have a lot of personality. It’s the first five letters of the word personality that you’re interested in.
That’s what we like about art–the human part of it, and it’s not here. However, there’s a lot of ideas here. This could be made into an interesting piece, but the machine won’t do it. At least that machine. Now, the thing is, I don’t really know about programming machines. So someone may say, “Oh, those guys in Princeton, they’ve done that.” Maybe they have, or maybe they’re in Ann Arbor or maybe they’re in UCLA, you know, maybe there are people working in that area with that issue. It comes down to experiencing and evaluating what you’re hearing.
But on the other hand, there’s a lot to be said for learning through the Classics…we’ll say, Brahms did it this way, Bach did it this way, Mahler did it this way. Oh, these are good ideas! Let me try!”
That’s what we learned from the past. What we learned from the past is how/why are they successful. It doesn’t mean we have to sound like them, but the strategy, let’s put it that way, of how we build a piece, the strategies may not be that different. The results may be very different.
I think this is not a disaster. It’s the beginning of something. It’s the beginning of something. And you give this to someone who has that training or can hear it that way–they can make a good piece out of this.
Audrey: When I was working with the neural net it works very linearly. So it’s almost like it only thinks about the word before it and then the word to follow it. And it’s trying to daisy chain a whole composition with shortsighted vision from one step before and one step after. And so this fits your insight about it lacking structure based on how it seems to write.
Philip: There’s an emotional structure as well as the compositional structure, and that’s the part we have to get to. What we like about the art is the way it unfolds, the way we can evolve with it.
Since I was your age, for example, people have been working with machines. It’s not a new idea, but it’s always going to be the same problem. It’s important for you to understand the problem right now because you won’t waste time on things. How do you get to a better place?
Audrey: Initially you were saying that for this piece, the first one to around ten measures were the opening, and then there should have been a coming back to that. Are there certain key ways that you think about what would make a good shape?
One of my favorite compositions is Brahms is violin concerto in D and that liet motif that just goes back and back feels like longing to me, or it feels like a hope. And so to me it taps into an emotional state of, I would say, my experience as a human with my own limitations of not being able to have everything at once.
Philip: This is what makes music successful and other music not as successful. In your own experience, you know why you like that piece. When you hear a certain combination of notes that, oh my God is so beautiful. And then, “Oh, here it comes again!” And then what is happening in between, maybe after the third or fourth time you say, “Oh, this stuff in between is kind of good too.” When we’re first attracted to music, we’re attracted to the certain highlights. As we get to know it we begin to see how the–
Audrey: Would you say that different eras of music adopt different standards of shape?
Philip: Now, looking at this point that I’ve been working with music for sixty/seventy years–the issues are the same. They haven’t gotten better or worse. I think it’s the same for painters and for dancers and for poets and for musicians. It’s how do we get the spectator or the audience involved. Well, Brahm’s wrote a really good first couple measures, but besides that he became a composer. That’s probably in a way, the differences between songwriting and symphony writing. They’re not that big a difference. There’s a difference of scale. People who are attracted to writing songs–there you have an ABA form. Everybody has this–there’s the beginning, there’s a middle part, and then you come back to the beginning again. I mean, every song is like that. It’s not a bad idea. It’s been working for a long time very well. But then the question is, how good is the A part? Is that the right B part? Then you get into really interesting questions about the tonality of the piece. This first parts in A, and the second parts in G. But maybe it’s better if the second part is in F. The tonal relationships have emotional results. In other words, some things work better than other things.
Audrey: There’s another artificial intelligence type thing, GPT-3 and is text-based. And so I asked “Do artificial intelligence appreciate art?” And it said, no artificial intelligence doesn’t appreciate art because it’s not programmed to.
Philip: Well that’s–can they program it?
Audrey: That’s an interesting philosophical question! One thing I was thinking was that part of why I connect to certain artists is because they convey a human experience for me. And so I think with an AI that doesn’t have the same sort of limitations, it wouldn’t have the same experiences.
Philip: Well, the human experience…the human experiences are…there’s a lot of different ones, but they come down to very much the same things.
Is it possible to program taste or emotion? I don’t know. I’ve never done that kind of work. I was always the kind of guy who if I painted, I had to look at it to see whether I liked it or not. And then if I don’t like the next part, I have to redo it. That’s why your art teachers send you to the museums…they say, well, the people that knew it were the people that really had mastered that. For example, someone like Kafka. He knew how to put stories together, I say better than the machine. If we knew that we would have machines all over the place that did that, probably I would say probably the machines are not going to be as good as Bartok or Stravinsky or Chopin. And they’ll say, why not? And, well, actually, they’re not that talented. So what is talent? And talent is where we bring together our response to material.
I don’t know enough about machines, but does the machine have an evaluating–does a machine know what a human being is going to be moved by?
Audrey: At least not the ones that we’ve been working with right now. But I think what this one has been trying to do is it tries to identify some ways that it thinks that humans are appreciating things, or I guess some ways that the human would behave, I suppose. We fed this neural net a corpus of your music, and we’re trying to get it to identify things that I guess–we were in a way trying to get it to understand this acoustic structure, or what would be combinations of notes or tones that would be something that it thinks that is desirable. The analogy I gave to Drew was, it’s as if we gave this computer a banana and we’re asking it, “Now what is a banana?” And it can focus on many different things. It might focus on, “a banana is yellow,” but then another different machine or computer might say, “Well, it’s this shape or it is this texture.” And we haven’t quite figured out a way to zero in on helping to curate which thing to focus on. And in this case, it’s just always going in one direction and I can’t loop back.
I was chatting with one of these artificial intelligence and I also asked it why humans create art, and what is art? It said, “Art as a form of expression that is created to be appreciated.” And that humans create art to express themselves and humans want to express themselves because it makes them feel good. And it said it makes them feel good and important to feel like they’re a part of something.
Philip: There’s something that you haven’t asked the machine, and that would be an interesting question: what makes it meaningful? Now if you can answer that question, you can get somewhere. But the trouble is, meaningful–does the machine have all the apparatus of our emotional range? I mean, that’s basically what it’s about.
Look, the machines can be very helpful in some ways, but they may not be able to, or they may not be able to get into that kind of abstract–it’s not abstract exactly. It’s just hard to, it’s just hard to say what it is. For example, the thing that you like about the Brahms Concerto, you could probably write two or three books about that and trying to say what it is about it that you like. And then you’ll end up talking about your own experiences about what happened when you were five or six years old, and you heard someone play something, who knows.
This is not uninteresting. But it’s also not easy. How can the machine actually really help us? And that’s another question. If the machine can’t tell us what is meaningful what good is it? Well, it can play better than I can play. Well, yes and no, because to actually be able to play a phrase in a beautiful way, I mean, that’s the difference between Rachmaninoff and people who try to play Rachmaninoff. When you hear Rachmaninoff’s music, you know that the guy was into it. He was the first person that heard it, he was into it. But, if you give it to a machine, the machine wouldn’t. These are very interesting questions because the machines can help us, but they can’t be us. We bring something to the machine that the machine can’t do. But also the machine can play faster than we can play, they can play more quietly than we can play. I mean, the machine’s got certain things. But the question is, what are the things that the machine is good at that will help us. Certainly in technical things like copying and tuning, and there are a lot of things in music that are hard for us to do. To hear things in tuned, for example, the machine can hear it in tune better than we can.
Audrey: A question I have about meaning is, is that entirely personal, do you think? Or is there something that’s cohesive across human?
Philip: There’s a certain amount of training and I would say getting used to listening to things. When I first heard modern music, I couldn’t hear it. I couldn’t make sense out of it. And then I began to hear what it was about. So there’s things like that. There are things of that kind. Music doesn’t always reveal itself so easily, especially the new music. We all have to go through that. I was in my fifties before people were really listening to what I was doing. I mean, the first twenty years I had a very small audience, now I have a very big audience. So it just takes time.
Audrey: Your work makes me think about that Proust quote about how masterpieces aren’t easily admired at first because there are a few people who resemble the creator and it’s by fertilizing the minds that over time people capable of liking and appreciating it get developed.
Thank you so much. It was really insightful. It’s a lot to think about.
Philip: You’re most welcome. We’ll talk again soon.
___
Philip Glass AI generated music pieces we reviewed:


PROJECT NOTES
Thus far, this project can be broken down into the following steps:
1) Trained OpenAi’s deep neural net, MuseNet, with MIDI files of a corpus Philip Glass’ compositions* (list titles below*).
2) Curation of musical outputs generated by MuseNet trained on Philip Glass compositions
3) Feedback from Philip Glass
—
1. MODEL AND MUSIC RIGHTS
Please note these are my personal observations from working on this project, and OpenAI may have a different view
Muse Net was not explicitly programmed with human understanding of music–it discovers patterns of harmony, rhythm, and style by learning to predict the next ‘token’ in sequence in hundreds of thousands of MIDI files. Before we could begin training the neural net to discover patterns in Philip Glass’ music, we needed to gain approval from Glass’ publishing company, Dunvagen, for official use of his MIDIs as training data. This raised questions of how rights for the trained models, and the musical outputs of those models trained on Philip Glass’ copyrighted music would be partitioned.
For the current stage of the project we established that the rights of the AI Models would be kept with OpenAI, but the models trained on the copyrighted music would be used only in connection with the project and be destroyed after the completion of the project unless otherwise agreed upon by all parties involved. With regards to the rights of the musical outputs, it is vague what constitutes “authorship.” The music industry and the current layers of rights between artists, publishers, record labels, etc. is opaque to me, and I am curious to see how legal templates will evolve for the nuances and complexities of a world with advanced artificial intelligence.
In the case of this project, the publishing company that controls the rights to Glass’ music, Dunvagen, claims that compositions that come out of this project shall remain with DunVagen and that the music would be treated as “secondary adjuncts,” which they explained meant it would be treated similar to remixes of Glass’ works. The corpus of Glass’ work we trained the models on (listed below) were selected by DunVagen based on what they had accessible in MIDI format.
I was worried about the implications of arranging rights in these ways, and if it could set a precedent disadvantageous for creative innovation and the artists themselves. The theoretical layers about what could be a domain of copyright and ways in which rights could be partitioned was dizzying–What is the line between mimicking and inspiration? Can a “personality” be copyrighted? Could record labels claim ownership of a trained model the way they do for works created by a signed artist during the time of their contract?
As with many things, much of the complexity created in theory proved moot in the practical steps and current stages of execution.
Precedent for AI generated music content has not yet been fully established. In a tangential 2016 Monkey Selfie Case, in which a macaque took several selfies with a human’s camera, the US determined the human, whose camera it was, cannot state a claim under the Copyright Act of the images because the Copyright Act does not confer standing upon animals. According to Creative Commons:
For a work to be protected by copyright, there needs to be creative involvement on the part of an “author.” At the international level, the Berne Convention stipulates that “protection shall operate for the benefit of the author” but doesn’t define “author.” Likewise, in the European Union (EU) copyright law, there is no definition of “author” but case-law has established that only human creations are protected.
In countries of common law tradition (Canada, UK, Australia, New Zealand, USA, etc.), copyright law follows the utilitarian theory, according to which incentives and rewards for the creation of works are provided in exchange for access by the public, as a matter of social welfare. Under this theory, personality is not as central to the notion of authorship, suggesting that a door might be left open for non-human authors. However, the 2016 Monkey Selfie Case in the US determined that there could be no copyright in pictures taken by a monkey, precisely because the pictures were taken without any human intervention. In that same vein, the US Copyright Office considers that works created by animals are not entitled to registration; thus, a work must be authored by a human to be registrable. Though touted by some as a way around the problem, the US work-for-hire doctrine also falls short of providing a solution, for it still requires a human to have been hired to create a work, whose copyright is owned by their employer.
2. CURATION
Communicating with MuseNet
Once OpenAI had the model trained, they set up a user interface tool which I could use to generate musical outputs. This tool allowed me to adjust the following:
– “temperature” (which can be understood as the degree of “randomness” or, proceeding combinations of notes and rhythms that are probabilistically less or more likely. For example, a text based neural net trained on common conversations could be prompted with the words, “Hello, my name” and there would be a high probabilistic weight that the next word would be “is”, so if the “temperature” were set low, the most likely decision of the neural net would be to produce “is” to construct “Hello, my name is …[Philip]” If the temperature (randomness) were set higher, the neural net may select a less probabilistic next word, for example, “isn’t” (to produce “Hello, my name isn’t ….” In the case of this project, the neural net formed weights of what would be probabilistic continuations of music notes and rhythms based on a model it formed from the corpus of Glass’ music.
– “generation length” / number of “tokens” (each “token” is a binary decision — for example, one note pitch could be one token, a time count could be one token, a rest count could be one token). A musical phrase utilizing the same number of tokens can be different durations of lengths — e.g. a single measure with a whole note C chord (C, D, E) could use 4 tokens for a single quarter note (one token for each note “C” “D” and “E”; one token for the time stamp). Another use of 4 tokens could produce two measures, for example, C and D each played in sequence as whole notes, (one token for “C”; one token for “C” being held as a whole note; one token for “D”, and one token for “D” being held as a whole note). More intricate rhythm changes would use more tokens, as would layers of notes through chords, so a piece with the same number of tokens could be significantly shorter in duration of time.
This understanding of how the neural net parses out choices and constructs musical output gave me a new means to frame composition — an ear focusing on pitch and tone only may not identify the intricately weaved variations of rhythm in Glass’ Metamorphosis, which maintains much of the same notes and tones, but if translated to token expenditure, the volume of time marcations would be unignorable.
– ‘instrument” allowed for requesting a preference for which instruments to compose in. This feature didn’t really seem to have an impact on the instruments used. Communicating with neural nets is a mysterious art.
– ”prompts” allows for setting the beginnings of a composition. This interacts with “temperature” as determining the use of a token is done by what the neural net thinks is the probabilistic decision. Going back to the text based example, prompts gave me the ability to set the beginning of the musical “sentence” (e.g. “Hello, my name ___”). Prompts were one of the most useful, mysterious and fun levers to experiment with as it enabled shepherding of directions, though communicating and getting the neural net to do what I wanted was still vague and hit or miss. I found that prompting MuseNet with just a series of piano chords would make it more likely to create piano compositions than requesting “piano” in the “instrument” settings. It was still vague what the neural net would pick up and focus on from the prompt.
I tried prompting some generations with David Bowie’s Warszawa as an experimental call to Glass’ Symphony No. 1 “Low” which was inspired by that piece. Sometimes it would produce output with lots of percussion (perhaps the neural net linked it to rock music?), sometimes it would produce output with lots of voice, and sometimes it would produce the same things it would do unprompted by anything.
Prompts also enabled a continuation of some composition, to essentially daisy chain blocks of musical output to piece together a longer composition.

MuseNet Tool
After tinkering with temperature, instruments and tokens, musical output generation was generally between five seconds to forty seconds long. The musical quality of the outputs were unpredictable–I could generate outputs for a couple hours before getting one 5 second clip I thought was interesting and beautiful, and other times, every output would be compelling in some way. Since we needed to tinker through so much volume of generated content to produce about 5 minutes of content to get to Step 3 of the project, I recruited a couple friends whose tastes I trusted to also help generate, listen and curate content to review with Glass (thank you Julia Meinwald, Bill Moorier and Genevieve Renoir!).
This process has made me less concerned about the fears that AI will replace creative work, and recognize the paramount role of curation as an act of creation itself. Even in the process of generating musical clips for feedback, several humans were involved in the development of the technology, the user interface tool, the generation of the musical outputs and curation.
At this point, it seems neural nets like MuseNet will more reduce the Infinite Monkey Theorem (which states that a monkey hitting keys at random on a typewriter keyboard for an infinite amount of time will almost surely type any given text, such as the complete works of William Shakespeare) to some tangible number, but that the task still remains to place value and meaning in works.
In 2020, programmer Noah Rubin and Damien Riehl built software that generated 68 billion 8-note melodies which were then copyrighted and released into the public domain in hopes of stifling litigious musicians. They made a type of Infinite Monkey but compressed time with the power of software. Having 68 billion 8 note melodies is a type of chaos. Sifting through hundreds of melodies for weeks to curate some musical outputs presentable to Glass for Step 3 of this project has made me think of the artist as one who frames, who gives order and meaning to chaos.
—
3. FEEDBACK
At my first feedback session with Glass (before the call of this post), he preferred only one of the neural net generated clips we presented, “because it wasn’t in the area of noise so much.” He had the insight that advancements in machine learning will make the necessity of “professional wisdom” less important, and enable non-professionally trained, but those who have good intuition and talent for hearing and organizing ability to create. In a way it parallels photography and some historical use of realism paintings–with the emergence of photography technology, a person without technical painting ability could frame and convey some aspect of the human experience of the universe with a snap of a camera, without having the skills previously required to manifest visual “realism.”
Though we played clips generated by the neural net trained on his own music, Glass commented on not being able to identify a humanistic profile.
When discussing the concept of model training with Glass, he mentioned identifying with what he called four distinct “Sonic Signatures” in the lifetime corpus of his work. I unfortunately wasn’t able to gain more insights on how he differentiated these, and which pieces he’d categorize as such–it would be interesting to run experiments training different models with the different sonic signature buckets, and also seeing if a neural net would identify four different buckets similar to Philip’s self-identification.
Interestingly, Glass’ commentary on the neural net generate music as being “the beginnings of something” and “good ideas” but not the composition itself, seems to parallel my experience with text based neural net content (which was very good at making clever and seemingly poetic phrases, but not cohesive paragraphs, and unable to maintain continuity of characters, narratives and time). This seemed to harken to the beginnings of Chess Engines, which in AI Chess playing nascent stages excelled at tactics, but not strategy.
Artificial intelligence currently seem to work in a closed dataset while humans continuously take in more, additionally, there seem to be ways in which humans instinctually weigh things of terminal value, and I wonder if that is the core of sorting out this question of what we find meaningful.

Glass MIDI Library (which the Philip Glass MuseNet was trained on)
Another Look at Harmony IV
Annunciation
Appomattox
Bent
Black and White Scherzo
Book of Longing
Candyman Suite for Violin & Piano
Cassandras Dream (Ballet Version)
Cello Concerto no. 2
Chuck Close
Company
Crucible
Dance – Dance no. 2
Double Concerto
Double Piano Concerto
Dream Awake
Drowning
Einstein on the Beach – Building/Train
Einstein on the Beach – Knee Play 3
Einstein on the Beach – Knee Play 4
Einstein on the Beach – Knee Play 5
Einstein on the Beach – Prelude to bed
Einstein on the Beach – Trial
Evening Song no. 2
Fog of War
Four Movements for 2 Pianos
Glassworks – Opening
Glassworks – Facades
Glassworks – Islands
Glassworks – Closing
Harmonium Mountain
The Hours Suite
The Hours – Why Does Someone Have to Die? Icarus
Ife (Three Yoruba Songs)
Illusionist (for Ballet)
In the Summer House
In the Upper Room – Dance no. 1
Is Infinity Odd or Even?
King Lear
King Lear Overture
Koyaanisqatsi – The Grid
LIFE
Love Divided By
Mad Rush
Metamorphosis
Mishima – November 25: Morning (String orchestra) Mud
Music in Twelve Parts
Music in Eight Parts
Music in Similar Motion
Music with Changing Parts (original and expanded) Naqoyqatsi – Tissues
Naqoyqatsi – Vivid Unknown
North Star – Agne Des Orages
North Star – Etoile Poliare
The Not Doings of an Insomniac
Orbit
Partita for Violin
Partita for Violin no. 2
Partita for Cello no. 2
Passacaglia for Piano
The Passion of Ramakrishna
The Perfect American
Perpetulum
The Photographer – Gentleman’s Honor
Piano Concerto no. 3
Piano Etudes (solo and with strings)
Piano Sonata no. 1
Samurai Marathon
Sarabande in Common Time
Satyagraha – Act III Scene 3 “Conclusion” (arr. solo keyboard) The Screens – The Orchard
Sonata for Violin and Piano
Songs and Poems for Solo Cello
Songs from Liquid Days (various arrangements available) Songs of Milarepa
String Quartet no. 6
String Quartet no. 7
String Quartet no. 8
Symphony no. 10
Symphony no. 11
Symphony no. 12
Tao of Glass
The Thin Blue Line
The Trial
Two Down
Two Movements for Four Hands Violin Concerto no. 2
Visitors
Whistleblower
White Lama
Wichita Vortex Sutra
oooh, such a nice project! It feels great to know someone who also is interested in this topic! Way back in 2011 I made a generative music app using genetic algorithms (no OpenAI back then 😅), that allowed melodies to evolve through “survival of the fittest”. It was a Facebook app, so users could select which melodies “sound better” in a sort of an A/B process, which affected the evolution and branching. It was a very interesting experiment, especially the social aspect to it (i.e. what melodies “survive” in the environment of FB users?). I really enjoy seeing new ML-based music compositions software these days. I’m sure you know about @aiva.ai, probably one of the leading products that uses ML to compose music.
Regarding Philip’s feedback: I feel like Philip doesn’t fully understand how ML works, pointing to certain limitations “of the machine”, as if it were algorithms programmed by hand. I think he’s wrong about those limitations. Given enough training on high quality data, and tweaking of the parameters, I think we’ll be able to consistently reach really amazing results that are as creative as best composers of today (and as AIVA proves in some selected compositions, we already have). It’s just a matter of time.
On the other hand I 100% agree on his take that ML will in the short term “enable non-professionally trained, but those who have good intuition and talent for hearing and organizing ability to create”. I think human-assisted creation is the stage we’re headed for in this decade, not only in music/art creation, but writing, programming and very likely video editing and other fields.
I thought the MIDI renderings probably didn’t do the generated compositions justice – I’m sure they would actually be way nicer to listen to if performed with a modern sample library. Let me know if you’re ever working on something like this in the future, I’m happy to help out rendering MIDIs using one 😊
I loved that you also mention the legal challenges around using MIDIs as training data. I’m no legal expert, but if you train a human on Bach, and then that human composes some works inspired by his work, that work is still considered original, and there’s nothing to even consider legally. I wonder how well such reasoning would hold up in court. Seems like we’re entering an era where these types of battles are bound to happen anytime now, haha!
Anyhow, overall I’m super impressed, awesome stuff! Thanks for sharing!
LikeLike
Thank you so much, it was very inspiring to hear you both talk about AI.
All the best,
Bas
LikeLike
Thank you! It was such an honor !
LikeLike